Dmca Complaint To Google
sender
submitter
to request access and see full URLs.
Copyright claim #2
DescriptionHIGH LEVEL INVESTING FOR DUMMIES – by MLADJENOVIChttps://www.wiley.com/en-us/High+Level+Investing+For+FOR DUMMIES-p-x000899473Original URLs: No copyrighted URLs were submitted. Allegedly Infringing URLs:
to request access and see full URLs.
Copyright claim #3
Kind of Work:UnspecifiedDescriptionSTATISTICAL ANALYSIS WITH R FOR DUMMIES – by SCHMULLERhttps://www.wiley.com/en-us/Statistical+Analysis+with+R+For+FOR DUMMIES-p-x001007505Original URLs: No copyrighted URLs were submitted. Allegedly Infringing URLs:
to request access and see full URLs.
Copyright claim #4
DescriptionMODEL-BASED SYSTEM ARCHITECTURE – by WEILKIENSOriginal URLs:
to request access and see full URLs.
Copyright claim #5
DescriptionMATERIALS FOR INTERIOR ENVIRONMENTS – by BINGGELIOriginal URLs:
to request access and see full URLs.
Copyright claim #6
DescriptionBRITISH SIGN LANGUAGE FOR DUMMIES – by CITY LITOriginal URLs:
to request access and see full URLs.
Copyright claim #7
DescriptionMETRIC PATTERN CUTTING FOR CHILDREN’S WEAR AND BABYWEAR – by ALDRICHOriginal URLs:
to request access and see full URLs.
Copyright claim #8
DescriptionCOMPUTATIONAL BIOLOGY: A HYPERTEXTBOOK – by KELLEYOriginal URLs:
to request access and see full URLs.
Copyright claim #9
DescriptionPERFORMING DATA ANALYSIS USING IBM SPSS – by MEYERSOriginal URLs:
The Role Of Big Data In Investing
Note: Separate multiple email address with a comma or semicolon.
Your Name:
Observations and views from investment professionals across GSAMs Quantitative Investment Strategies team on the role of big data in potential investments.
- Osman Ali, Portfolio Manager, Quantitative Investment Strategies, GSAM
- Takashi Suwabe, Portfolio Manager, Quantitative Investment Strategies, GSAM
- Dennis Walsh, Portfolio Manager, Quantitative Investment Strategies, GSAM
What Is Your Approach To Big Data In Emerging Markets
Dennis Walsh: We feel that the information asymmetry in emerging markets may create opportunities for data-driven investors like ourselves. A lack of available data is a sign of mispricing and uncertainty, and investors who are diligent enough to analyze and uncover potential opportunities in this environment may be rewarded. With 4,000 companies in the emerging market universe, spanning 23 countries across 6 continents, it can be a challenge to capture and digest vast amounts of disparate information, especially since the quality of data or reporting governance standards in some of these countries is lacking. Our experience and sophisticated techniques make us well-positioned to act in this space and analyze potential investments without necessarily requiring us to have analysts locally based around the world. This centralization of data processing is more scalable and allows us to cover a wider breadth of companies when compared to traditional methods.
Although certain information has been obtained from sources believed to be reliable, we do not guarantee its accuracy, completeness or fairness. We have relied upon and assumed without independent verification, the accuracy and completeness of all information available from public sources.
THIS MATERIAL DOES NOT CONSTITUTE AN OFFER OR SOLICITATION IN ANY JURISDICTION WHERE OR TO ANY PERSON TO WHOM IT WOULD BE UNAUTHORIZED OR UNLAWFUL TO DO SO.
Read Also: How To Start An Investment Newsletter
Can You Explain Your Investment Philosophy And How Access To Big Data Has Impacted How You Invest
Osman Ali: We are focused on creating data-driven investment models that can objectively evaluate public companies globally through fundamentally-based and economically-motivated investment themes. These models have historically utilized a large set of company-specific data like publicly available financial statement, as well as market data like prices, returns, volumes, etc. With the growth and availability of non-traditional data sources such as internet web traffic, patent filings and satellite imagery, we have been using more nuanced and sometimes unconventional data to help us gain an informational advantage and make more informed investment decisions.
Machine Learning And Big Data In Quantitative Investing
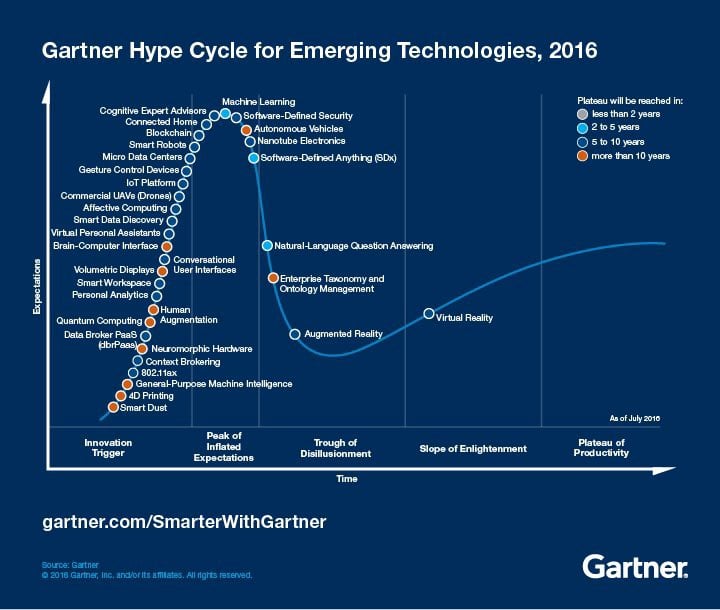
Finance professionals apply machine learning and big data techniques to solve investment problems and improve investment performance.
Read this white paper to learn how you can use MATLAB® for:
- Asset allocation and optimization
- Sentiment analysis with natural language processing
- Outlier and fraud detection
- Financial forecasting and price prediction
Recommended Reading: How To Invest In A Company Before It Goes Public
Big Data And Machine Learning In Quantitative Investment
La disponibilidad es orientativa y puede sufrir modificaciones
Añadir a mis favoritos
- Debes estar identificado para añadir artículos a tu lista de favoritos. Puedes hacerlo desde aquí
Compartir
Súmate hoy mismo al programa de puntos TROA
© 2022 DELSA – TROA Librerías. Todos los derechos reservados. Aviso LegalGráficos diseñados por Freepik.com
Esta web utiliza cookies propias y de terceros para mejorar nuestros servicios mediante el análisis de sus hábitos de navegación. Puede obtener más información aquí o cambiar la configuración.
How Do Portfolio Managers Interact With The Models That Are Analyzing Data And Making Recommendations
Takashi Suwabe: Data is the basis of our investment model, but the research and portfolio construction processes still require human judgement. Portfolio managers exercise their judgment when selecting the data and analytics that we use in investing, and also when reviewing and approving each trade in every portfolio. This is to ensure that all portfolio positions make sensethat they are economically intuitive and appropriately sized given current market conditions. We do not have a computer in the corner simply shooting out trades with no human interaction.
We are researching new factors and analytics that have an impact on stock prices, and our portfolio managers drive that research. Research success for us is not finding a new stock to invest in, but rather finding a new investment factor that can help improve the way we select stocks. Investment factors should be fundamentally-based and economically-motivated, and the data enables us to empirically test our investment hypotheses. We would never work in the opposite directionobserving relationships in the data that we would seek to justify or explain after-the-fact.
Also Check: How To Easily Invest In Stocks
What Kinds Of Boundaries Are You Pushing Now And What Do You See As The Future Of Big Data
Dennis Walsh: Active management has always been about uncovering opportunities before they are priced in by the broader market. The exponential growth in data is fueling our investment decisions and research agenda. Were seeking to push boundaries by moving beyond conventional data sources and leveraging alternative forms of data to gain an informational edge.
Today, were able to process more data more quickly, in an effort to uncover insights and connections that arent as obvious to other investors. Given new data availability and the development of machine learning techniques to learn quickly from such data, we are only at the beginning of this Data Revolution that we believe is transforming every industry globally.
How Has Your Technology And Infrastructure Evolved To Keep Up With Big Data
Dennis Walsh: New data storage technologies have created the infrastructure needed to capture, analyze and make informed decisions from new forms of real-time data. For example, the growth of distributed databases, where data is stored across several platforms in place of a single platform via a centralized database, allows for highly-scalable parallel processing of vast amounts of data. This can decrease processing time by several orders of magnitude for many applications. Unstructured data storage also allows for greater flexibility in onboarding and retrieving data from non-traditional sources and in managing large amounts of text-based information.
Recommended Reading: Best Bank Accounts For Investment Clubs
What Types Of Data Are You Analyzing And How Does It Differ From What You Were Looking At Before The Data Revolution
Takashi Suwabe: We identify strong businesses with attractive valuations, positive sentiment and a strong connection with positive themes that are trending in the markets. The types of data we analyze now are quite a bit more expansive than what we used 10 years ago. In the past, computers could only analyze structured data, or data that is easily quantifiable and organized in a set form. New technologies allow us to analyze unstructured data, or data that is not as easily quantified. These innovations enable us to interpret information from a much wider variety of sources, including language, images and speech for the first time.
Access to new types of data, along with the ability to capture and process that data quickly, has given us new ways to capture investment themes such as momentum, value, profitability and sentiment.
The Secret To Automating Machine Learning Life Cycles
he automation revolution businesses are seeing in the 21st century is comparable to the industrial revolution of the 18th century. Automation refers to the controlling of a system or process using minimal human assistance. Similar to how machines in factories forever changed efficient production, digital automation is now finding even more ways to work faster and smarter.
The life cycle of machine learning is defined as a circular process involving a pipeline development, training phase, or inference phase. Data scientists and engineers use ML to develop and train models in various applications. They are also able to make quick data-informed decisions without much human intervention.
Companies are able to save significant time and resources by automating manual processes. This reduces overhead and time-to-market while allowing data scientists to focus on creative tasks. Knowing the secrets to automate machine learning life cycles gives companies the ability to more efficiently gather data and make informed decisions. Digital tools are the secret to maximizing the impact of ML. The models that are being developed will also be used in various different ways that will either generate more revenue, make processes more efficient, or solve complex business problems.
Breakdown of the machine learning lifecycle
The importance of automating machine learning
AI Integration
About the Author
Sign up for the free insideBIGDATA newsletter.
Join us on Twitter:
Join us on Facebook:
Don’t Miss: What Is Investment Risk Management